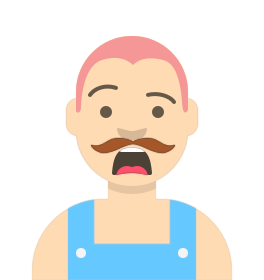
Reputation
Badges 1
979 × Eureka!I think my problem is that I am launching an experiment with python3.9 and I expect it to run in the agent with python3.8. The inconsistency is from my side, I should fix it and create the task with python3.8 with:task.data.script.binary = "python3.8" task._update_script(convert_task.data.script)
Or use python:3.9 when starting the agent
I have a mental model of the clearml-agent as a module to spin my code somewhere, and the python version running my code should not depend of the python version running the clearml-agent (especially for experiments running in containers)
Should I open an issue in github clearml-agent repo?
then print(Task.get_project_object().default_output_destination)
is still the old value
Yes, perfect!!
This works well when I run the agent in virtualenv mode (remove --docker
)
Hi AgitatedDove14 , that’s super exciting news! 🤩 🚀
Regarding the two outstanding points:
In my case, I’d maintain a client python package that takes care of the pre/post processing of each request, so that I only send the raw data to the inference service and I post process the raw output of the model returned by the inference service. But I understand why it might be desirable for the users to have these steps happening on the server. What is challenging in this context? Defining how t...
Hi CostlyOstrich36 , most of the time I want to compare two experiments in the DEBUG SAMPLE, so if I click on one sample to enlarge it I cannot see the others. Also once I closed the panel, the iteration number is not updated
What I put in the clearml.conf is the following:
agent.package_manager.pip_version = "==20.2.3" agent.package_manager.extra_index_url: ["
"] agent.python_binary = python3.8
AgitatedDove14 I have a machine with two gpus and one agent per GPU. I provide the same trains.conf to both agents, so they use the same directory for caching venvs. Can it be problematic?
I am using an old version of the aws autoscaler, so the instance has the following user data executed:echo "{clearml_conf}" >>/root/clearml.conf ... python -m clearml_agent --config-file '/root/clearml.conf' daemon --detached --queue '{queue}' --docker --cpu-only
super, thanks SuccessfulKoala55 !
Will the from clearml import Task
raise an error if no clearml.conf exists? Or only when actual features requiring to define the server (such as Task.init
) will be called
Thanks! With this I’ll probably be able to reduce the cluster size to be on the safe side for a couple of months at least :)
but according to the disks graphs, the OS disk is being used, but not the data disk
Seems like it just went unresponsive at some point
But you might want to double check
SuccessfulKoala55 Since 2 hours I get 504 errors and I cannot ssh into the machine. AWS reports that instance health checks fail. Is it safe to restart the instance?
There’s a reason for the ES index max size
Does ClearML enforce a max index size? what typically happens when that limit is reached?
SuccessfulKoala55 I am looking for ways to free some space and I have the following questions:
Is there a way to break-down all the document to identify the biggest ones? Is there a way to delete several :monitor:gpu and :monitor:machine time series? Is there a way to downsample some time series (eg. loss)?
Well, as long as you’re using a single node, it should indeed alleviate the shard disk size limit, but I’m not sure ES will handle that too well. In any case, you can’t change that for existing indices, you can modify the mapping template and reindex the existing index (you’ll need to index to another name, delete the original and create an alias to the original name as the new index can’t be renamed...)
Ok thanks!
Well, as long as you use a single node, multiple shards offer no sca...
can it be that the merge op takes so much filesystem cache that the rest of the system becomes unresponsive?
The number of documents in the old and the new env are the same though 🤔 I really don’t understand where this extra space used comes from
Here is (left) the data disk (/opt/clearml) and right the OS disk
it also happens without hitting F5 after some time (~hours)
Here is the console with some errors
Yes, I set:auth { cookies { httponly: true secure: true domain: ".clearml.xyz.com" max_age: 99999999999 } }
It always worked for me this way
"Can only use wildcard queries on keyword and text fields - not on [iter] which is of type [long]"
if I want to resume a training on multi gpu, I will need to call this function on each process to send the weights to each gpu