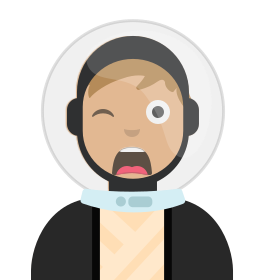
Reputation
Badges 1
2 × Eureka!Nice find! I'll pass it through to the relevant devs, we'll fix that right up 🙂 Is there any feedback you have on the functionality specifically? aka, would you use alias give what you know now or would you e.g. name it differently?
Please do, if you find any more issues (due to my shitty code or otherwise 😄 ) let me know and I'll fix 'em!
I'm still struggling to reproduce the issue. Trying on my own PC locally as well as on google colab yields nothing.
The fact that you do get tensorboard logs, but none of them are captured by ClearML means there might be something wrong with our tensorboard bindings, but it's hard to pinpoint exactly what if I can't get it to fail like yours 😅 Let me try and instal exactly your environment using your packages above. Which python version are you using?
Based on the screenshot of you package versions, it does seem like tensorboard is not installed there. We depend on that, because every scalar logged to tensorboard is captured in ClearML too. My guess would be that maybe you installed tensorboard in e.g. the wrong virtualenv.
However, you do say you tested it with Tensorboard and even then it didn't work. In that case, are the scalars correctly logged to tensorboard? You should be able to easily check this by doing a run, and then launching...
@<1558986839216361472:profile|FuzzyCentipede59> Would you mind sharing how you're running the training? i.e. a minimal code example so we can reproduce the issue?
Hi UnevenBee3 , the OptimizerOptuna class should already be able to prune any bad tasks, provided the model itself is iteration-based (so no SVM etc. need iterations for early stopping). You can read our blogpost here: https://clear.ml/blog/how-to-do-hyperparameter-optimization-better/
Yeah, I do the same thing all the time. You can limit the amount of tasks that are kept in HPO with the save_top_k_tasks_only
parameter and you can create subprojects by simply using a slash in the name 🙂 https://clear.ml/docs/latest/docs/fundamentals/projects#creating-subprojects
@<1523701949617147904:profile|PricklyRaven28> Please use this patch instead of the one previously shared. It excludes the dict hack :)
Hi @<1546303293918023680:profile|MiniatureRobin9> !
Would you mind sending me a screenshot of the model page (incl the model path) both for the task you trained locally as well as the one you trained on the agent?
Thanks! I know that you posted these locations before in text, I just wanted to make sure that they are the ones I was thinking. It seems like the model isn't properly uploaded to the clearml server. Instead, it's saving only the local path to the model file.
Normally that's what the output_uri=True
in the Task.init(...)
call is for, but it seems there is a bug that's not uploading the model.
Would you mind testing out [manual model uploading](https://clear.ml/docs/latest/docs/clea...
Hi CourageousKoala93 ! Have you tried https://clear.ml/docs/latest/docs/references/sdk/task#set_comment by any chance? There's a description field under the info tab 🙂
I tried answering them as well, let us know what you end up choosing, we're always looking to make clearml better for everyone!
I added a reply to one of the issues 🙂 edit: answered both issues, the third issue is the same as your question here on slack.
Sure! This is an example of running a custom model. It basically boils down to defining a preprocess, process and postprocess
function. Inside the process
function can be anything, including just a basic call to huggingface to run inference 🙂
I have not tested this myself mind you, but I see no reason why it wouldn't work!
In fact, I think even Triton itself supports running on CPU these days, so you still ...
That wasn't my intention! Not a dumb question, just a logical one 😄
As I understand it, vertical scaling means giving each container more resources to work with. This should always be possible in a k8s context, because you decide which types of machines go in your pool and your define the requirements for each container yourself 🙂 So if you want to set the container to use 10.000 CPUs feel free! Unless you mean something else with this, in which case please counter!
Usually those models are Pytorch right? So, yeah, you should be able to, feel free to follow the Pytorch example if you want to know how 🙂
Sorry, I jumped the gun before I fully understood your question 🙂 So with simple docker compose file, you mean you don't want to use docker-compose-triton.yaml
file and so want to run the huggingface model on CPU instead of Triton?
Or do you want to know if the general docker compose version is able to handle a huggingface model?
To be honest, I'm not completely sure as I've never tried hundreds of endpoints myself. In theory, yes it should be possible, Triton, FastAPI and Intel OneAPI (ClearML building blocks) all claim they can handle that kind of load, but again, I've not tested it myself.
To answer the second question, yes! You can basically use the "type" of model to decide where it should be run. You always have the custom model option if you want to run it yourself too 🙂
Hey! So several things here:
As per the plotly docs, you have to give which axis you want to format, in your example plotly can't know. If you look closely to their example, you'll see it's a nested dict, with the key being 'xaxis' Your numpy data has x values of 1 2 3, but your extra layout has values 1 3 4 which don't match. Plotly took the first element of each subarray to be the x value.
If we fix these 2 things, your example becomes:
` task.logger.report_line_plot('this_is_the_title',
...
The above works for me, so if you try and the command line version does not work, there might be a bug. Please post the exact commands you use when you try it 🙂
effectively making us lose 24 hours of GPU compute
Oof, sorry about that, man 😞
Hi Alejandro! I'm running the exact same Chromium version, but haven't encountered the problem yet. Are there specific parameter types where it happens more often?
Hi ExuberantParrot61 ! Can you try using a wildcard? E.g. ds.remove_files(dataset_path='folder_to_delete/*')
The scheduler just downloads a dataset using the ID right? So if you don't upload a new dataset, the scheduler is just downloading the dataset from the last known ID then. I don't really see how that could lead to a new dataset with it's own ID as the parent. Would you mind explaining your setup in a little more detail? 🙂
Hello!
What is the usecase here, why would you want to do that? If they're the same dataset, you don't really need lineage, no?
Hey ExasperatedCrocodile76 ! Thanks for checking back in and letting me know 😄 Glad I could help!