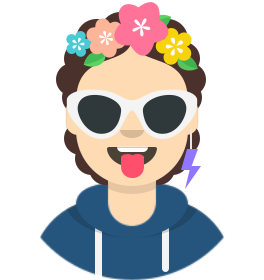
Reputation
Badges 1
90 × Eureka!How we resolved this issue is by developing a package to deal with the connecting and querying to databases. This package is then used inside the task for pulling the data from the data warehouse. There is a devops component here for authorising access to the relevant secret (we used SecretsManager on AWS). The clearml-agent instances are launched with role permissions which allow access to the relevant secrets. Hope that is helpful to you
I don't even know if I have a valid concern for this. Just a little worried as airflow is accessible by more departments than just DS, which could result in some disasters
Haha no not that much, I was just trying to play around with removing tasks etc, and didn't want to remove tasks created by co-workers.
Out of interest, is there a reason these are read-only? The code for these tasks is on github right?
We are planning on using airflow as the orchestration, but that may not fit your needs. I would say that the tool of choice is highly context specific.
We will be using airflow to trigger clearml-defined pipelines based on 'trigger' events, such as degradation in model performance, error alerts (e.g. at the data transformation task) etc.
We are planning to use Airflow as an extension of clearml itself, for several tasks:
we want to isolate the data validation steps from the general training pipeline; the validation will be handled using some base logic and some more advanced validations using something like great expectations. our training data will be a snapshot from the most recent 2 weeks, and this training data will be used across multiple tasks to automate the scheduling and execution of training pipelines periodically e...
are the envs named after the worker enumeration? e.g. venv-bulds-0 is linked to worker 0?
ohhh ok. so I can actually remove this if those workers are no longer in use
ECR access should be enabled as part of the role the agent instance assumes when it runs a task
Oh great, thanks! Was trying to figure out how the method knows that the docker image ID belongs to ECR. Do you have any insight into that?
Sorry, just revisiting this as I'm only getting around to implementation now. How do you pass the ECR container ID to the defined task?
yes it does, but that requires me to manually create a new agent every time I want to run a different env no?
And how will it know that the container is on ECR instead of some other container repository?
That's a good question, which I don't have an answer to 😅 I was hoping to be able to store the config file in some kind of secrets vault, and authenticating via some in-memory trace or so
Oh, that may work. Is there any docs/demos on this?
Locally or on the remote server?
I am struggling to fill in the values for the template. Some are obvious, others are not
I think there is more complexity to what I am trying to achieve, but this will be a good start. Thanks!
I will need to log data set ID, transformer (not the NN architecture, just a data transformer), the model (with all hyperparameters & metadata) etc. and how all things link
Sounds good to me. Thanks Martin 🙂
Did the shell script route work? I have a similar question.
It's a little more complicated because the index URL is not fixed; it contains the token which is only valid for a max of 12 hours. That means the ~/.config/pip/pip.conf
file will also need to be updated every 12 hours. Fortunately, this editing is done automatically by authenticating AWS codeartefact in the command line by logging in.
My current thinking is as follows:
Install the awscli
- pip install awscli
(c...
One question - you can also set the agent.package_manager.extra_index_url
, but since this is dynamic, will pip install still add the extra index URL from the pip config file? Or does it have to be set in this agent config variable?
Awesome, thank you. I will give that a try later this week and update if it worked as expected! May finally solve my private dependencies issue 😂
By script, you mean entering these two lines separately as a list for that extra_docker_shell_scripts
arugment?
So how do I ensure that artefacts are uploaded in the correct bucket from within clearml?
As in an object from memory directly, without having to export the file first. I thought boto3 can handle this, but looking at the docs again, it doesn't look like it. File-like objects is their term, so maybe an export is required
Reason I am asking is because we have servers with large RAM capacity, but minimal storage capacity, meaning that objects held in memory can sometimes surpass storage capacity if export is required
This is included as part of the config file at ~/clearml.conf
on the clearml-agent
extra_docker_shell_script: [ "apt-get install -y awscli", "aws codeartifact login --tool pip --repository data-live --domain ds-15gifts-code", ]
Not sure how to get a log from the CLI but I can get the error from the clearml server UI, one sec
Using SSH credentials - replacing https url '
' with ssh url '
' Replacing original pip vcs 'git+
' with '
` '
Collecting py_db
Cloning ssh://@github.com/15gifts/py-db.git (to revision 851daa87317e73b4602bc1bddeca7ff16e1ac865) to /tmp/pip-install-zpiar1hv/py-db
Running command git clone -q 'ssh://@github.com/15gifts/py-db.git' /tmp/pip-install-zpiar1hv/py-db
2021-12-08 15:56:31
ERROR: Repository not found.
fatal: Could not read from remote repository.
Please...