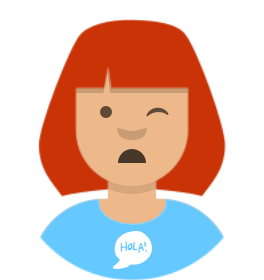
Reputation
Badges 1
16 × Eureka!mmm... Since they are saved "automatically" without my intervention i am not sure i can know to which "training" (hyperparams and training set combination) each one belongs to.
Interesting proposal. Why use the "post" callback and not the "pre" callback?
I guess i need to do something like the following after the task was created:
` from clearml.binding.frameworks import WeightsFileHandler
def callback(_, model_info):
model_info.name = "my new name"
return model_info
WeightsFileHandler.add_pre_callback(callback) `
We do upload the final model manually.
I was just wondering if i can make the autologging usable. Right now when i don't know (at least in the web ui) on which hyperparameter set the model was trained on and on which data (full train set, one of the cv combinations) i have no use for these uploaded models.
The object would be enough. The problem is that I currently don't have a way to get them "from outside".
I actually just tried to use model_info.local_model_path
assuming its the pickled model file path(debug prints showed its a single file and not a directory) but it failed pickle.load
In my case its the xgboost model object , but yes.
There were two types of model upload. The first one is clearml automatic upload when GridSearchCV was running.
The second one is manual by us when GridSearchCV finished and we got a final model. We "manually" uploaded this model and had control over its name.
My question was about the automatically uploaded models. Those that were uploaded by clearml client.
SweetBadger76 Thanks for your detailed response. I was able to receive the graph with the layout i wanted (the left image you sent). However the problem with this approach is that when comparing two experiments it will show me two separate graph which makes it harder to compare individual bars.
I use sklearn's GridSearchCV (not clearml HPO)
so all models are part of the same experiment and has the experiment name in their name.
I don't see any hyper parameter in the model name.
about 1, It uploads the models as artifacts and i also see them in the web UI in the model list.
The document is not clear enough but if understand your answer to disable only the model upload and registration i should pass something like'xgboost': False
or 'xgboost': False, 'scikit': False
?
about 2, I refer to the names of the models.
Thanks!
How can i obtain the actual trained model class inside the callback function ? basically i need to know what are its hyperparameters.
I am using the Task.init()
approach
and then running RandomizedSearchCV
(not using ClearML's HPO).
Trying that now passing verbose=3
to sklearn's class.
I can see the verbose message on the clearml's console tab while the search runs so this is a kind of a poor's man solution to my problem buy may be enough for now.
Regarding using clearml HPO, will it create multiple experiments on the UI for each tested hyperparameters set or would i be able to see all those trials in a ...
AgitatedDove14 Thanks for you help. I've opened an issue.
SweetBadger76 Thanks for your elaborate example. Its very helpful!
AgitatedDove14 Your suggestion is probably what I wanted, however it does not seem to change the orientation.
I tried adding extra_layout={"orientation":'h'}
as the last parameter to the report_histogram but I still see the "vertical" (default) orientation. Should I pass some other parameter value to extra_layout
or should i report a bug?
Won't help since the problem is that i don't know the model args (its hidden inside GridSearchCV implementation and i can't access it).
Related to that, I was able to unpickle the file that you upload as a model (MODEL URL in the UI model list). It turns out to be a joblib pickled file but the content seem strange: a numpy array of the form [0,1,2,3,...] (so each cell contains its offset).
Is that normal or a possible bug?
We are running a hyperparameter tuning (using some cv) which might take a long time and might be even aborted unexpectedly due to machine resources.
We therefore want to see the progress. what hyperparameters set were tested and what was their results summary metrics (i.e avg and stddev of ROCAUC across all cv's).