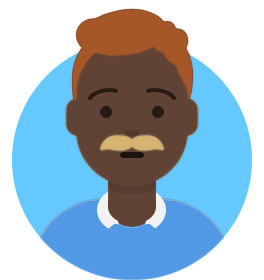
Reputation
Badges 1
371 × Eureka!With online learning, my two main concerns are that the training would be completely stochastic in nature, I would not be able to split the data into train test splits, and that it would be very expensive and inefficient to train online.
It'll be labeled in the folder I'm watching it.
I get what you're saying. I was considering training on just the new data to see how it works. To me it felt like that was the fastest way to deal with data drift. I understand that it may introduce instability however. I was curious how other developers who have successfully managed to set up continuous training deal with it. 100% new data, or a ratio between new and old data. And if it is the latter, what should be the case, which should be the majority, old data or new data?
Understandable. I mainly have regular image data, not video sequences so I can do the train test splits like you mentioned normally. What about the epochs though? Is there a recommended number of epochs when you train on that new batch?
Would you know what the pros would be to learning online other than the fact that the incoming data is as close to the current distribution of data based on time as possible for us. Also would those benefits worth it to train online?
Should I just train for 1 epoch? Or multiple epochs? Given I'm only training on the new batch of data and not the whole dataset?
Thank you, I'll start reading up on this once I've finished setting up the basic pipeline
It's basically data for binary image classification, simple.
My main query is do I wait for it to be a sufficient batch size or do I just send each image as soon as it comes to train
Lastly, I have asked this question multiple times, but since the MLOps process is so new, I want to learn from others experience regarding evaluation strategies. What would be a good evaluation strategy? Splitting the batch into train test? that would mean less data for training but we can test it asap. Another idea I had was training on the current batch, then evaluating it on incoming batches. Any other ideas?
Sorry for the late response. Agreed, that can work, although I would prefer a way to access the data by M number of batches added instead of a certain range, since these cases aren't interchangeable. Also a simple thing that can be done is that you can create an empty Dataset in the start, and then make it the parent of every dataset you add.
Another issue I'm having is I ran a task using clearml-task and did it using a repo. It runs fine, when I clone said task however and run it on the same queue again, it throws an error from the code. I can't seem to figure out why its happening.
I plan to append the checkpoint to a list, when the len(list) > N, I'll just pop out the one with the highest loss, and delete that file from clearml and storage. That's how I plan to work with it.
Creating a new dataset object for each batch allows me to just publish said batches introducing immutability.
Anyway, in the docs, there is a function called task.register_artifact()
Considering I don't think the function itself requires Venv to run normally but in this case it says it can't find venv
Well I'm still researching how it'll work. I'm expecting it to not be very good and will make the model learning very stochastic in nature.
I plan to instead at the training stage, instead of just getting this model, use Dataset.squash, to get previous M datasets merged together.
This should introduce stability in the dataset.
Also this way, our model is trained on a batch of data multiple times but only for a few times before that batch is discarded. We keep the training data fresh for co...
because those spawned processes are from a file register_dataset.py , however I'm personally not using any file like that and I think it's a file from the library.
I ran a training code from a github repo. It saves checkpoints every 2000 iterations. Only problem is I'm training it for 3200 epochs and there's more than 37000 iterations in each epoch. So the checkpoints just added up. I've stopped the training for now. I need to delete all of those checkpoints before I start training again.
Basically since I want to train AI Models right. I'm trying to set up the architecture where I can automate the process from data fetching to model training, and need GPU for training.