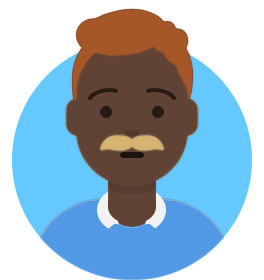
Reputation
Badges 1
371 × Eureka!Can you spot something here? Because to me it still looks like it should only create a new Dataset object if batch size requirement is fulfilled, after which it creates and publishes the dataset and empties the directory.
Once the data is published, a dataset trigger is activated in the checkbox_.... file. which creates a clearml-task for training the model.
The scheduler is set to run once per hour but even now I've got around 40+ anonymous running tasks.
apparently it keeps caliing this register_dataset.py script
I initially wasn't able to get the value this way.
wrong image. lemme upload the correct one.
There's a whole task bar on the left in the server. I only get this page when i use the ip 0.0.0.0
I basically had to set the tag manually in the UI
Let me try to be a bit more clear.
If I have a training task in which I'm getting multiple ClearML Datasets from multiple ClearML IDs. I get local copies, train the model, save the model, and delete the local copy in that script.
Does ClearML keep track of which data versions were gotten and used from ClearML Data?
I then did what MartinB suggested and got the id of the task from the pipeline DAG, and then it worked.
CostlyOstrich36
adding tags this way to a Dataset object works fine. This issue only occured when doing this to a model.
Anyway I restarted the triton serving engine.
I'm using clear-ml agent right now. I just upload the task inside a project. I've used arg parse as well however as of yet, I have not been able find writable hyperparameters in the UI. Is there any tutorial video you can recommend that deals with this or something? I was following https://www.youtube.com/watch?v=Y5tPfUm9Ghg&t=1100s this one on youtube but I can't seem to recreate his steps as he sifts through his code.
I've been having this issue for a while now :((
Well yeah, you can say that. In add function step, I pass in a function which returns something. And since I've written the name of the returned parameter in add_function_step, I can use it, but I can't seem to figure out a way to do something similar using a task in add_step
let me check
It does to me. However I'm proposing a situation where a user gets N number of Datasets using Dataset.get, but uses m number of datasets for training where m < n. Would it make sense to only log the m datasets that were used for training? How would that be done?
I feel like they need to add this in the documentation 😕
I'm not using decorators. I have a bunch of function_steps followed by a normal task step, where I've passed a base_task_id.
I want to check the value of one of the functional steps, and if it holds true, I want to execute the task step otherwise I want the pipeline to end there, since the task step is the last one.
It works this way. Thank you.
I'll look into it. Thank you everyone.
I got to that conclusion I think yeah. Basically, can access them as artifacts.
Ok this worked. Thank you.
AnxiousSeal95 I just have a question, can you share an example of accessing an artifact of a previous step in the pre execute callback?
I checked and it seems when i an example from git, it works as it should. but when I try to run my own script, the draft is in read only mode.
Thank you, this is a big help. I'll give this a go now.
You can see there's no task bar on the left. basically I can't get any credentials to the server or check queues or anything.