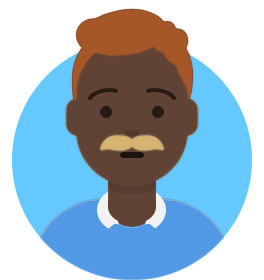
Reputation
Badges 1
371 × Eureka!Collecting idna==3.3
Using cached idna-3.3-py3-none-any.whl (61 kB)
Collecting importlib-metadata==4.8.2
Using cached importlib_metadata-4.8.2-py3-none-any.whl (17 kB)
Collecting importlib-resources==5.4.0
Using cached importlib_resources-5.4.0-py3-none-any.whl (28 kB)
ERROR: Could not find a version that satisfies the requirement jsonschema==4.2.1 (from -r /tmp/cached-reqsm1gu3664.txt (line 19)) (from versions: 0.1a0, 0.2, 0.3, 0.4, 0.5, 0.6, 0.7, 0.8.0, 1.0.0, 1.1.0, 1.2.0, 1.3.0, 2.0...
So I just published a dataset once but it keeps scheduling task.
I just copied the commands in order from the page and pasted them. All of the linux ones specifically.
Honestly anything. I tried looking up on youtube but There's very little material there, especially which is up to date. It's understandable given that ClearML is still in beta. I can look at courses / docs. I just want to be pointed in the right direction as to what I should look up and study
Thanks for the help. I'll try to continue working on the vm for now.
I shared the error above. I'm simply trying to make the yolov5 by ultralytics part of my pipeline.
AgitatedDove14 Alright I think I understand, changes made in storage will be visible in the front end directly.
Will using Model.remove, completely delete from storage as well?
the storage is basically the machine the clearml server is on, not using s3 or anything
I need to both remove the artifact from the UI and the storage.
up to date with https://fawad_nizamani@bitbucket.org/fawad_nizamani/custom_yolov5 ✅
Traceback (most recent call last):
File "train.py", line 682, in <module>
main(opt)
File "train.py", line 525, in main
assert os.path.isfile(ckpt), 'ERROR: --resume checkpoint does not exist'
AssertionError: ERROR: --resume checkpoint does not exist
Since that is an immediate concern for me as well.
when i pass the repo in clearml-task with the parameters, it runs fine and finishes. Basically when I clone and attempt the task again, I get the above assert error I don't know why.
so when I run the task using clearml-task --repo and create a task, it runs fine. It runs into the above error when I clone the task or reset it.
for which I basically forked it for myself. and made it accept clearml dataset and model ids to use.
I've basically just added dataset id and model id parameters in the args.
Is there a difference? I mean my use case is pretty simple. I have a training and it basically creates a lot of checkpoints. I just want to keep the n best checkpoints and whenever there are more than N checkpoints, I'll delete the worst performing one. Deleted both locally and from the the task artifacts.
Another issue I'm having is I ran a task using clearml-task and did it using a repo. It runs fine, when I clone said task however and run it on the same queue again, it throws an error from the code. I can't seem to figure out why its happening.
I ran a training code from a github repo. It saves checkpoints every 2000 iterations. Only problem is I'm training it for 3200 epochs and there's more than 37000 iterations in each epoch. So the checkpoints just added up. I've stopped the training for now. I need to delete all of those checkpoints before I start training again.
I plan to append the checkpoint to a list, when the len(list) > N, I'll just pop out the one with the highest loss, and delete that file from clearml and storage. That's how I plan to work with it.
And given that I want have artifacts = task.get_registered_artifacts()
Currently every 2000 iterations, a checkpoint is saved, that's just part of the code. Since output_uri = True, it gets uploaded to the ClearML server.
How do I go about uploading those registered artifacts, would I just pass artifacts[i] and the name for the artifact?
I just assumed it should only be triggered by dataset related things but after a lot of experimenting i realized its also triggered by tasks, if the only condition passed is dataset_project and no other specific trigger condition like on publish or on tags are added.
'dataset' is the name of my Dataset Object
Well I'm still researching how it'll work. I'm expecting it to not be very good and will make the model learning very stochastic in nature.
I plan to instead at the training stage, instead of just getting this model, use Dataset.squash, to get previous M datasets merged together.
This should introduce stability in the dataset.
Also this way, our model is trained on a batch of data multiple times but only for a few times before that batch is discarded. We keep the training data fresh for co...
I'm still a bit confused around the fact that since my function runs once per hour, why are there indefinitely growing anonymous tasks, even after i've closed the main schedulers.
I'll test it with the updated one.
The scheduler is set to run once per hour but even now I've got around 40+ anonymous running tasks.
Shouldn't I get redirected to the login page if i'm not logged in instead of the dashboard? 😞