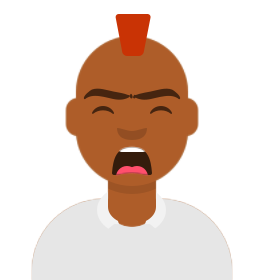
IntriguedBat44
3
Questions,
8
Answers
Active since 10 January 2023
Last activity
2 years ago
Reputation
0
Badges 1
8 × Eureka!When I run experiments I set CUDA_VISIBLE_DEVICES to some integer to only make that device available to the main process (as is common). I can verify that th...
4 years ago
Hi everyone, if I want to run a script that has Trains tracking statements in it but just this time I want to disable all logging, how would I go about that?
4 years ago
Hey! Is there a way to ignore the spammy output of progressbars like progressbar2 and tqdm in the captured log in Trains?
4 years ago