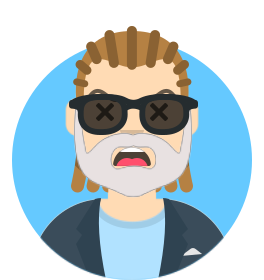
Reputation
Badges 1
25 × Eureka!ColossalDeer61 FYI all is fixed now π
Which means you currently save the argument after resolving and I'm looking to save them explicitly so the user will not forget to change some dependencies.
That is correct
I'm looking to save them explicitly so the user will not forget to change some dependencies.
Hmm interesting point. What's the use case for storing the values before the resolving ?
Do we want to store both ?
The main reason for storing the post resolve values, is that you have full visibility to the actual...
(I'll make sure it is added to the docstring because apparently it was not there
RattySeagull0 I think you are correct, python 3.6 is the installed inside the docker. Is it important to have 3.7 ? You might need another docker (or change the installation script and install python 3.7 inside)
i keep getting an failed getting token error
MiniatureCrocodile39 what's the server you are using ?
Notice the order here:Task.add_requirements("tensorflow") task = Task.init(...)
clearml doesnβt do any βmagicβ in regard to this for tensorflow, pytorch etc right?
No π and if you have an idea on how, that will be great.
Basically the problem is that there is no "standard" way to know which layer is in/out
let me check a sec
the services queue (where the scaler runs) will be automatically exposed to new EC2 instance?
Yes, using this extra_clearml_conf
parameter you can add configuration that will be passed to the clearml.conf
of the instances it will spin.
Now an example to the values you want to add :agent.extra_docker_arguments: ["-e", "ENV=value"]
https://github.com/allegroai/clearml-agent/blob/a5a797ec5e5e3e90b115213c0411a516cab60e83/docs/clearml.conf#L149
wdyt?
using this is it possible to add to requirements of task with task_overrides?
Correct, but you will be replacing (not adding) requirements
ChubbyLouse32 could it be the configuration file is not passed to the agent machine itself ?
(were you able to run anything against this internal server? I mean to connect to it from code, clearml/cleamrl-agent) ?
The latest RC (0.17.5rc6) moved all logs into separate subprocess to improve speed with pytorch dataloaders
Hi TrickySheep9
You should probably check the new https://github.com/allegroai/clearml-server-helm-cloud-ready helm chart π
https://github.com/allegroai/clearml-server-helm-cloud-ready
Is there any way to debug these sessions through clearml? Thanks!
Yes this is a real problem, AWS does not allow to get the data very easily...
Can you check the AWS console, see what you have there ?
In theory this should have worked.
Maybe we you are missing some escaping for the "extra_vm_bash_script" ?
I'm hoping the console output will tell us
Hi JealousParrot68
do tasks that are created through create_function_task run the entry_script again instead of just the pure function
Basically they will run the code until the "create_function_task" call, but never after. We are working on adding a decorator to a function, making it a "standalone" script, is this what you actually need ?
This is the reason you are getting an error π
Basically the session asks the agent to setup a new SSH server with credentials on the remote machine, this is not an issue inside a container, as this is an isolated environment, but when running in venv mode the User running the agent is not root, hence it cannot spin/configure an SSH server.
Make sense ?
it's in the docker image, doesn't the git clone command run in the container
Then this should have worked.
Did you pass in the configuration: force_git_ssh_protocol: true
https://github.com/allegroai/clearml-agent/blob/e93384b99bdfd72a54cf2b68b3991b145b504b79/docs/clearml.conf#L25
JitteryCoyote63 not yet π
I actually wonder how poplar https://github.com/pallets/click is ?
Bummer... that seems like a bit of an oversight tbh.
There is never a solution for those, unless the helm chart "knows" something about the server before spinning it the first time, which basically means a predefined access-key, I do not think we want that π
tell me please, does the agent always create a virtual environment?
Yes, but it inherits from the container preinstalled system environment
is it possible to make the agent run the script in an already prepared docker container without creating a virtual environment in the container?
You can set the CLEARML_AGENT_SKIP_PIP_VENV_INSTALL=1
environment variable
Hi ShallowCormorant89
Can you verify the http link is valid? Can you download it from code on your machine (i.e. not via an agent), maybe 8081 port is blocked from the agent machine to the server?
However, the pipeline experiment is not visible in the project experiment list.
I mean press on the "full details" in the pipeline page
Okay verified, it won't work with the demo server. give me a minute π
Hi ImpressionableRaven99
Yes, it is π
Call this one before task.init, and it will run offline (at the end of the execution, you will get a link to the local zip file of the execution)Task.set_offline(True)
Then later you can import it to the system with:Task.import_offline_session('./my_task_aaa.zip')
Hi PungentLouse55
it depends on the trains-server version you are running.
If the trains-server >= 0.16 then you have to add "Args/" prefix. If you are running an older version, then you should not add any prefix.
At the moment I'm querying by paging through the tasks as you recommended, and then filtering with standard python list-comprehension filters...Which is less than ideal.
At least let's do that better:
Use Task._query_tasks:Task._query_tasks(order_by=['-started'], page_size=10, page=0, only_fields=['id', 'started'])
You will get "lighter" objects returned, then you can filter them with code (but the request will be a lots faster)
SuccessfulKoala55 any suggestion on improving that ?