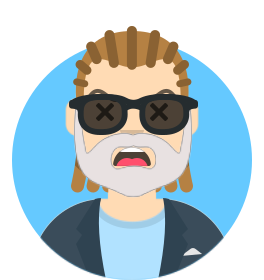
Reputation
Badges 1
25 × Eureka!No worries, just found it. Thanks!
I'll make sure to followup on the GitHub issue for better visibility 🙂
Unfortunately that is correct. It continues as if nothing happened!
oh dear, let me make sure this is taken care of
And thank you for the reproduce code!!!
Hi PanickyMoth78
Yes i think you are correct, this looks like gs throttling your connection. You can control the number of concurrent uploads with max_worker=1
https://github.com/allegroai/clearml/blob/cf7361e134554f4effd939ca67e8ecb2345bebff/clearml/datasets/dataset.py#L604
Let me know if it works
WittyOwl57 I think this is a great idea, can you open a feature issue on GitHub so this is not forgotten ?
BTW: regardless, if you have time to upgrade to the new the azure package upgrade, it will be great 🙂 this is on our to do list for a while, but since not a lot of users complained it got pushed ...
I still see things being installed when the experiment starts. Why does that happen?
This only means no new venv is created, it basically means install in "default" python env (usually whatever is preset inside the docker)
Make sense ?
Why would you skip the entire python env setup ? Did you turn on venvs cache ? (basically caching the entire venv, even if running inside a container)
from clearml import TaskTypes
That will only work if you are using the latest from the GitHub, I guess the example code was modified before a stable release ...
Hi ExcitedFish86
Of course, this is what it was designed for. Notice in the UI under Execution you can edit this section (Setup Shell Script). You can also set via task.set_base_docker
Hi WickedGoat98
A few background notions:
Docker do not store their state, so if you install something inside a docker, the moment you leave, it is gone, and the next time you start the same docker you start from the same initial setup. (This is a great feature of Dockers) It seems the docker you are using is missing wget. You could build a new docker (see the Docker website for more details on how to use a Dockerfile). The way trains-agent works in dockers is it installs everything you ne...
Okay, so basically set a template for the pod, specifying the docker image. Make sure you pass the correct trains-server configuration (i.e. api/web/file server addresses and credentials), and select the queue name the agent will listen to.
container image / details
https://hub.docker.com/r/allegroai/trains-agent
https://github.com/allegroai/trains-agent/tree/master/docker/agent
Full environment variable list to pass can be found here:
https://github.com/allegroai/trains-server/blob/...
WickedGoat98 sorry, I missed the thread...
that the trains.conf has to be located on the node running the trains-agent.
Correct 🙂
The easiest way to check is to see if you can curl to the ip:port from the docker.
If you fail it is probably the wrong IP.
the IP you need to use is the IP of the machine running the docker-compose (not the IP of the docker inside that machine).
Make sense ?
For example:agent.docker_preprocess_bash_script = [ "echo 'Binary::apt::APT::Keep-Downloaded-Packages \"true\";' > /etc/apt/apt.conf.d/docker-clean", "apt-get update", "apt-get install -y wget", "echo \"we have wget\"", ]
WickedGoat98 Basically you have two options:
Build a docker image with wget installed, then in the UI specify this image as "Base Docker Image" Configure the trains.conf
file on the machine running the trains-agent, with the above script. This will cause trains-agent
to install wget on any container it is running, so it is available for you to use (saving you the trouble of building your own container).With any of these two, by the time your code is executed, wget is installed an...
WickedGoat98
for such pods instantiating additional workers listening on queues
I would recommend to create a "devops" user and have its credentials spread across all agents. sounds good?
EDIT:
There is no limit on number of users on the system, so login as a new one and create credentials in the "profile" page :)
ReassuredTiger98 no, but I might be missing something.
How do you mean project-specific?
Could you test if this is working:
https://github.com/allegroai/clearml/blob/master/examples/reporting/matplotlib_manual_reporting.py
LOL totally 🙂
Basically it hooks into any torch.save function (monkey patching in realtime)
If you cannot change the "TrainerState" (i.e. inherit and pass it into the code)
you cloud also monkey-patch it, something like
` class OurTrainerState(TrainerState):
def init(...)
...
def load_from_json(cls, json_path: str):
super().load_from_json(json_path))
Task.current_task().upload_artifact(...)
trainer.state = OurTrainerState(trainer.state) `
but could you try with the latest RC?
is everything on the same network?
what do you mean? the same env for all components ? if they are using/importing exactly the same packages, and using the same container, then yes it could
The task pod (experiment) started reaching out to an IP associated with malicious activity. The IP was associated with 1000+ domain names. The activity was identified in AWS guard duty with a high severity level.
BoredHedgehog47 What is the pod container itself ?
EDIT:
Are you suggesting the default "ubuntu:18.04" is somehow contaminated ?
https://hub.docker.com/layers/library/ubuntu/18.04/images/sha256-d5c260797a173fe5852953656a15a9e58ba14c5306c175305b3a05e0303416db?context=explore
Is there any better way to avoid the upload of some artifacts of pipeline steps?
How would you pass "huge datasets (some GBs)" between different machines without storing it somewhere?
(btw, I would also turn on component caching so if this is the same code with the same arguments the pipeline step is reused instead of reexecuted all over again)
BTW: you can always set different config files by with an environment variable:CLEARML_CONFIG_FILE="path/to/cobfig/file