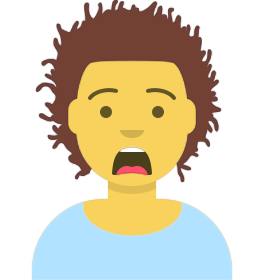
Reputation
Badges 1
84 × Eureka!I expect either 'var1' to be 'b' or - better - there to be log of the change, so that I would be able to see how the value changed over time.
No these are 3 different ways of building pipelines.
That is what I meant to say 🙂 , sorry for the confusion, @<1523701205467926528:profile|AgitatedDove14> .
@<1523701083040387072:profile|UnevenDolphin73> , your point is a strong one. What are clear situations in which pipelines can only be build from tasks, and not one of the other ways? An idea would be if the tasks are created from all kinds of - kind of - unrelated projects where the code that describes the pipeline does not ...
KindChimpanzee37 : First I went to the dataset and clicked on "Task information ->" in the right bottom corner of the "VERSION INFO". I supposed that is the same as what you meant with "right click on more information"? Because I did not find any option to "right click on more information". The "Task information ->" leads me to a view in the experiment manager. I posted the two screen shots.
PS: It is weird to me that the datamanager leads me to the experiment manager, specifically an experi...
KindChimpanzee37 , any idea 🙂 ?
@<1523701087100473344:profile|SuccessfulKoala55> : That is the link I posted as well. But this should be mentioned also at places where it is about about the external or non-external storage. Also it should be mentioned everywhere we talk about models or artifacts etc. Not necessarily in details, but at least with a sentence and a link.
KindChimpanzee37 , this time, I was away for a week 🙂 . I do not think, that I made the mistake you suggested. At the top of the script I wroteproject_name = 'RL/Urbansounds'
and then later
` self.original_dataset = Dataset.get(dataset_project=project_name, dataset_name='UrbanSounds example')
This will return the pandas dataframe we added in the previous task
self.metadata = Task.get_task(task_id=self.original_dataset.id).artifacts['metadata'].get() `
KindChimpanzee37 , I ensured that the dataset_name is the same in get_data.py and preprocessing.py and that seemed to help. Then, I got the error RuntimeError: No audio I/O backend is available.
, because of which I installed PySoundFile
with pip; that helped. Weirdly enough then, the old id error came back. So, I re-ran get_data.py and then preprocessing.py - this time the id error was gone again. Instead, I got `raise TypeError("Invalid file: {0!r}".format(self.name))
TypeError:...
But, I guess @<1523701070390366208:profile|CostlyOstrich36> wrote that in a different chat, right?
: What does
- The component code still needs to be self-composed (or, function component can also be quite complex)
Well it can address the additional repo (it will be automatically added to the PYTHONPATH), and you can add auxilary functions (as long as they are part of the initial pipeline script), by passing them to
helper_functions
mean? Is it not possible that I call code that is somewhere else on my local computer and/or in my code base? That makes thi...
Thank you I found the error.myPar = task.connect(myPar, name='from TaskParameters')
is required.
Do you mean "exactly" as in "you finally got it" or in the sense of "yes, that was easy to miss"?
@<1523701083040387072:profile|UnevenDolphin73> : How do you figure? In the past, my colleagues and I just shared the .zip
file via email / MS Teams and it worked. So I don't think so.
@<1537605940121964544:profile|EnthusiasticShrimp49> : The biggest advantage I see to split your code into pipeline components is caching. A little bit structuring your code, but I was told by the staff this should not one's main aim with ClearML components. What is your main take away for splitting your code into components?
My HPO on top of the pipeline is already working 🙂 I am currently experimenting on using the HPO in a (other) pipeline that creates two HPO steps (from the same funct...
@<1523701083040387072:profile|UnevenDolphin73> : Thanks, but it does not mention the File Storage of "ClearML Hosted Server".
@<1523701083040387072:profile|UnevenDolphin73> : From which URL is your most recent screenshot?
KindChimpanzee37 : Ok, will do. (More question from my side though. :-D) But I need to have pretty good idea before presenting our concept to the bosses.
Also, I could not find any larger examples on github about Model, InputModel, or OutputModel. It's kind of difficult to build a PoC this way... 😅
@<1523701070390366208:profile|CostlyOstrich36> : After more playing around, it seems that ClearML Server does not store the models or artifacts itself. These are stored somewhere else (e.g., AWS S3-bucket) or on my local machine and ClearML Server is only storing configuration parameters and previews (e.g., when the artifact is a pandas dataframe). Is that right? Is there a way to save the models completely on the ClearML server?
@<1523701070390366208:profile|CostlyOstrich36> : Thanks, where can I find more information on ClearML's model repository. I hardly find any in the documentation.
Also, that leaves the question open, what Model
is for. I described how I understand, the workflow should look like, but my question remains open...
@<1523701070390366208:profile|CostlyOstrich36> I read those, but did not understand.
I think now that here the documentation means the usage of connect
.
CostlyOstrich36 any ideas?
CostlyOstrich36 sure:[..]\urbansounds8k\venv\lib\site-packages\torchaudio\backend\utils.py:62: UserWarning: No audio backend is available. warnings.warn("No audio backend is available.") ClearML Task: overwriting (reusing) task id=[..] 2022-09-14 14:40:16,484 - clearml.Task - INFO - No repository found, storing script code instead ClearML results page:
`
Traceback (most recent call last):
File "[..]\urbansounds8k\preprocessing.py", line 145, in <module>
datasetbuilder = DataSe...
@<1523701083040387072:profile|UnevenDolphin73> : I do not get this impression, because during update_weights
I get the message
2023-02-21 13:54:49,185 - clearml.model - INFO - No output storage destination defined, registering local model C:\Users..._Demodaten_FF_2023-02-21_13-53-51.624362.model
As far as I understand, the workflow is like this. I define some model. Then I register it as an OutputModel. Then I train it. During training I save snapshots (not idea how, though) and then I save the final model when training is finished. This way the Model is a) connected to the task and b) available in the model store of ClearML.
Later, in a different task, I can load an already trained model with InputModel. This InputModel is read-only (regarding the ClearML model store), but I can ma...
Ok, I checked: A is terminated. This is not what I thought would happen and not what I intended with my documentation. I should clarify that.
I just see the website that I linked to. I am not sure what is meant by "python environment". I cannot make a screen shot, because I do not know where to look for this in the first place.