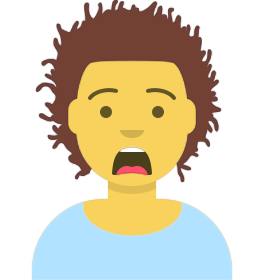
Reputation
Badges 1
84 × Eureka!@<1523701083040387072:profile|UnevenDolphin73> : I do not get this impression, because during update_weights
I get the message
2023-02-21 13:54:49,185 - clearml.model - INFO - No output storage destination defined, registering local model C:\Users..._Demodaten_FF_2023-02-21_13-53-51.624362.model
I am not sure if it the fact the name of the file ends with .model
is an issue - but that would be somewhat crazy design...
But, I guess @<1523701070390366208:profile|CostlyOstrich36> wrote that in a different chat, right?
@<1523701070390366208:profile|CostlyOstrich36> : Thanks, where can I find more information on ClearML's model repository. I hardly find any in the documentation.
Also, that leaves the question open, what Model
is for. I described how I understand, the workflow should look like, but my question remains open...
@<1523701070390366208:profile|CostlyOstrich36> : After more playing around, it seems that ClearML Server does not store the models or artifacts itself. These are stored somewhere else (e.g., AWS S3-bucket) or on my local machine and ClearML Server is only storing configuration parameters and previews (e.g., when the artifact is a pandas dataframe). Is that right? Is there a way to save the models completely on the ClearML server?
"using your method you may not reach the best set of hyperparameters."
Of course you are right. It is an efficiency trade-off of speed vs effectiveness. Whether this is worth it or not depends on the use-case. Here it is worth it, because the performance of the modelling is not sensitive to the parameter we search for first. Being in the ball-park is enough. And, for the second set of parameters, we need to do a full grid search (the parameters are booleans and strings); thus, this wo...
@<1523701205467926528:profile|AgitatedDove14> : "does that make sense ?" Not really.
"you do not need to automatically Add/Log/Track things into the Task in the current process." - I do not need to automatically do [...]? You mean I can do it automatically, but alternatively I can do it manually? Do you mean I use close
within a process to prevent automatic logging/adding/tracking? But, as far as I know, after I used close
I am not able to log etc. manually either. So...
"Mark...
How would you compare those to ClearML?
@<1523701070390366208:profile|CostlyOstrich36> , I am build a PoC, evaluating if we should use ClearML for our entire ML team and go Scale or Enterprise pricing. For that I need to know all/most capabilities and concepts of ClearML to see if ClearML is future-proof.
TL;DR: difficult to narrow it down, but we (amongst other things), we need a model store
Ok, I checked: A is terminated. This is not what I thought would happen and not what I intended with my documentation. I should clarify that.
@<1523701070390366208:profile|CostlyOstrich36>
My training outputs a model as a zip file. The way I save and load the zip file to make up my model is custom made (no library is directly used), because we invented the entire modelling ourselves. What I did so far:
output_model = OutputModel(task=..., config_dict={...}, name=f"...")
output_model.update_weights("C:\io__path\...", is_package=True)
and I am trying to load the model in a different Python process with
mymodel =...
Secondly, I do not understand this:
None says
Manually mark a Task as completed. This will close the running process and will change the Task’s status to Completed (Use this function to close and change status of remotely executed tasks). To simply change the Task’s status to completed, use task.close()
None says
Closes the current Task and cha...
Last point on component caching, what I suggest is actually providing users the ability to control the cache "function". Right now (a bit simplified but probably accurate), this is equivalent to hashing of the following dict:
{"code": "code here", "container": "docker image", "container args": "docker args", "hyper-parameters": "key/value"}
We could allow users to add a function that get's this dict and returns a new dict that will be used for hashing. This way we will e...
It means "The syntax for the file name, folder name or volume label / disk is wrong" somthing along those lines. The [...] is the directory path to my project, which I opened in PyCharm and from which I run the commands in the Python Console.
>pip show clearml
WARNING: Ignoring invalid distribution -upyterlab (c:\users\...\lib\site-packages)
WARNING: Ignoring invalid distribution -illow (c:\users\...\lib\site-packages)
Name: clearml
Version: 1.6.4
Summary: ClearML - Auto-Magical Experiment Manager, Version Control, and MLOps for AI
Home-page:
None
`Auth...
I am running it in the Python Console in PyCharm with Task.init. I get with the log:
ClearML Task: overwriting (reusing) task id=dfa2dff538d54c18ad97ea1593cbd357
2023-02-14 13:06:44,336 - clearml.Task - WARNING - Failed auto-detecting task repository: [WinError 123] Die Syntax für den Dateinamen, Verzeichnisnamen oder die Datenträgerbezeichnung ist falsch: '[...]\<input>'
ClearML results page: [None](https://app.clear.ml/projects/9acc061c880344a881790461a4baa837/experiments/dfa2dff538d54c1...
@<1523701083040387072:profile|UnevenDolphin73> , you wrote
Well, I would say then that in the second scenario it’s just rerunning the pipeline, and in the third it’s not running it at all
(I mean, in both the code may have changed, the only difference is that in the second case you’re interested in measuring it, and in the third,
you’re not, so it sounds like a user-specific decision).
Well, I would hope that in the second scenario step A is not rerun. Yes, in the third scen...
@<1523701205467926528:profile|AgitatedDove14> : In general: If I do not build a package out of my local repository/project , I cannot reference anything
from the local project/repository directly, right? I must make a package out of it, or I must reference it with the repo
argument, or I must reference respective functions using the helper_functions
argument. Did I get this right?
@<1523701205467926528:profile|AgitatedDove14> , you wrote
- Components anyway need to be available when you define the pipeline controller/decorator, i.e. same codebase
No you an specify a different code base, see here:
Is the code in this "other" repo downloaded to the agent's machine? Or is the component's code pushed to the machine on which the repository is?
If the second case is true: How is the other machine (on which the other repo is lying on) turned into an agent?