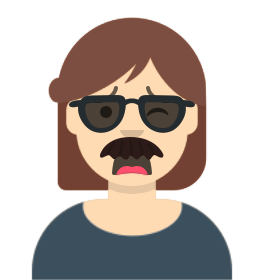
Reputation
Badges 1
32 × Eureka!if in the "installed packages" I have all the packages installed from the requirements.txt than I guess I can clone it and use "installed packages"
Because at the moment I'm having a problem with the s3fs package where I have it in my requirements.txt but the import manager at the entry point doesn't install it
After the agent finished installing the "requirements.txt" it will put back the entire "pip freeze" into the "installed packages", this means that later we will be able to fully reproduce the working environment, even if packages change (which will eventually happen as we cannot expect everyone to constantly freeze versions)
This would be perfect
"Pytorch Lightning need the s3fs " s3fs is not needed, let PL store the model locally and use "output_uri" to automatically upload the model to your S3 bucket.
So I can set output_uri = "s3://<bucket_name>/prefix" and the local models will be loaded into the s3 bucket by ClearML ?
No ok now I think I got how to use it, so "detect_with_pip_freeze" suppose that the instance launching remotely the clearml task has already all the packages installed inside pip and store them in the "installed packages". After this all the remote clearml-agents will install the packages included in "installed packages". Correct?
Yes it does 👍 Btw, at the moment I added import(s3fs) in my entry point and it's working, thank you!
Please let me know if my explanation is not really clear
Yes the workaround it's working 🙂
Ok now I noticed that If I change the value of the port inside the Hydra parameters section ( not the overrides) It does actually change also in the experiment. The overrides doesn't seem to be working
However, If I edit directly the OmegaConf in the UI than the port changes correctly. I'd still prefer to override the Args so I can change entire sub-configuration e.g. ['dataset=cifar']
to ['dataset=imagenet']
instead of having to change all the parameters inside the OmegaConf
I've just seen it is a know issue https://clearml.slack.com/archives/CTK20V944/p1611763839133700 . Has a new version been released meanwhile?
Hi AgitatedDove14 , I noticed that in the Hydra parameters section it is not possible to add as parameters keys string with dots: .(dot) $(dollar) and space are not allowed in parameter key.
However, it's very useful to add parameters with the dot to change something in a sub-configuration as, for example, training.max_epochs=10
. Do you think it's possible to allow this?
Hi AgitatedDove14 , thank you for your answer!
At the moment I can't configure both internal/external with the same dns. Before changing the server infrastructure, i'm trying a workaround where I upload the artifact with the internal file server path, and then I upload a string artifact which is the first artifact url where I replace the internal dns with the external dns, and use it to download the artifact from the UI.
Hi AgitatedDove14 , do you mean the the k8s glue autoscaler here https://github.com/allegroai/clearml-agent/blob/master/examples/k8s_glue_example.py ? If yes, I understood that this service deploys pods on the nodes in the cluster, but I'd prefer to have a new instance deployed for each new experiment and that it also terminates when no new experiments are queued
Hi AgitatedDove14 , FriendlySquid61 ! I managed to grant permission to the AWS autoscaler to spin instances using the instance profile as suggested by FriendlySquid61 . The instances are created and terminated correclty, however the new instances don't executed the queued task and shutdown immediately. I noticed that the clearml credential atself.web_server = Session.get_app_server_host()
self.api_server = Session.get_api_server_host()
` self.files_server = S...
AgitatedDove14 that seems like the best option. Once the aws autoscaler is inside a docker container I can deploy it inside a kube pod or a job. This, however, requires that I slightly modify the clearml helm chart with the aws-autoscaler deployment, right?