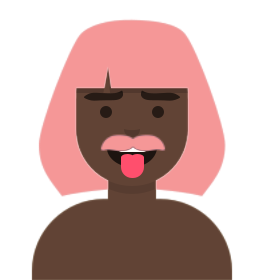
Reputation
Badges 1
75 × Eureka!I've poked around both the internal URL that Jupyter kernel is running on and some of the files in /sagemaker/.jupyter
but no luck so far - I can find plenty of kernel info, but not session
environ{'PYTHONNOUSERSITE': '0',
'HOSTNAME': 'gfp-science-ml-t3-medium-d579233e8c4b53bc5ad626f2b385',
'AWS_CONTAINER_CREDENTIALS_RELATIVE_URI': '/_sagemaker-instance-credentials/xxx',
'JUPYTER_PATH': '/usr/share/jupyter/',
'SAGEMAKER_LOG_FILE': '/var/log/studio/kernel_gateway.log',
'PATH': '/usr/local/sbin:/usr/local/bin:/usr/sbin:/usr/bin:/sbin:/bin:/tmp/miniconda3/condabin:/tmp/anaconda3/condabin:/tmp/miniconda2/condabin:/tmp/anaconda2/condabin'...
nice! Just tested it on my end as well, looks like it works!
lots of things like {"__timestamp__": "2023-02-23T23:49:23.285946Z", "__schema__": "sagemaker.kg.request.schema", "__schema_version__": 1, "__metadata_version__": 1, "account_id": "", "duration": 0.0007679462432861328, "method": "GET", "uri": "/api/kernels/6ba227af-ff2c-4b20-89ac-86dcac95e2b2", "status": 200}
As another test I ran Jupyter Lab locally using the same custom Docker container that we're using for Sagemaker Studio, and it works great there, just like the native local Jupyter Lab. So it's seemingly not the image, but maybe something to do with how Studio runs it as a kernel.
I additionally tried using a Sagemaker Notebook instance, to see if it was the kernel dockerization that Studio uses that was messing things up. But it seems to actually log less information from a Notebook instance vs Studio .

directly inside the notebook I can manually cause it to log the Hydra config, it just doesn't seem to autodetect if you're doing a manual call to Hydra Compose
My use case is running forecasting models in production across multiple businesses
I could just loop through and create separate pipelines with different parameters, but seems sort of inefficient. the hyperparameter optimization might actually work in this case utilizing grid search, but seems like kind of a hack
those look like linear DAGs to me, but maybe I'm missing something. I'm thinking something like the map operator in Prefect where I can provide an array of ["A", "B", "C"]
and run the steps outlined with dotted lines independently for each of those are arguments
But we're also testing out new models all the time, which are typically implemented as git branches - they run on the same set of inputs but don't output their results into production