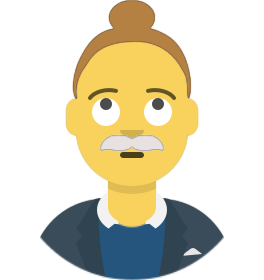
UpsetWalrus59
17
Questions,
32
Answers
Active since 09 May 2023
Last activity
one year ago
Reputation
0
Badges 1
31 × Eureka!Hi, I just started using ClearML and I really enjoy it - it seems much easier than other similar tools. I got today a bit mysterious message when running exp...
one year ago
Hi, it seems that Autorefresh stopped working for me. When checked it used to automatically update charts in the Scalars tab and now it is not happening (eve...
one year ago
Hi, what is the expect input of the series parameter of the logger.report_line_plot function? I am passing list of two lists (like [[1,2,3], [4,5,6]] ) to pl...
one year ago
Hi, could somebody please confirm if the output_uri parameter for creating Dataset in Azure is in the form: "azure://. blob.core.windows.net/ " ?
one year ago
Hi, After my model's iterations ended and task turned to Completed I wanted to still train the model further for more iterations. I found there is continue_l...
one year ago
Hi, I am considering different plans ClearML offers and it would be great if somebody could confirm if my understanding is correct. So for now what I underst...
one year ago
Hi, I added in my code task.ignore_requirements("pywin32") however this library is still getting added by ClearML to the packages (which causes error on Linu...
one year ago
Hi, after I create my clearml.conf file with clearml-agent init I edit the azure.storage field afterwards to put there account_name and account_key , is ther...
one year ago
Hi, I am able to execute the task locally, but the same task fails when executed on remote cloud machine, I think the error is due to the fact that local mac...
one year ago
Hi, I noticed that when I commit changes and not push them and try to run a job I am getting clearml_agent: ERROR: Failed cloning repository. and advice to M...
one year ago
Hi, what would be the best practice for using the Datasets when testing different features? Should I just add new Dataset each time I try new feature to the ...
one year ago
Hi, what could be possible reason for changing hyperparameter from UI not working? The same hyperparameter changed in the UI in the cloned experiment gives d...
one year ago
Hi, I noticed that my experiments fail if I try to clone them and enqueue them after I already pushed another commits (following the commit of the cloned exp...
one year ago
Hi, I am having trouble with comparing plotly plots from different experiments. The plots, when you look at them within one experiment look fine (attaching s...
one year ago
Hi, I am getting line 57, in get_local_copy if helper.base_url == "file://": AttributeError: 'NoneType' object has no attribute 'base_url' when trying to run...
one year ago
Hi, what could be the reason for my Task.init taking close to 10min? See below screenshot of example run which took around 8min.
one year ago
Hi, is there any way to download all the experiments including their metrics, hyperparameters and so on?
one year ago