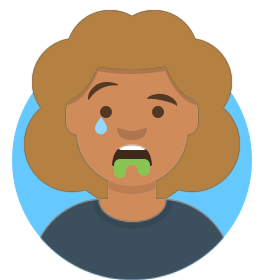
Reputation
Badges 1
16 × Eureka!thank you!
I'll add a volume mount to the services-agent container, and from what I understand that will become the template it uses?
is this the structure of the file?
None
or is it the "dot" syntax (like what shows up in the console when the task executes / your snippet)?
maybe an important note: I mounted the same cache directory for the agents.
then back to CLI, updated the pipeline to point the tasks to the new queue. run it, shows up in the UI (same container as default worker, just replicated w a new docker-compose and CMD to point to the new queue).
the dataset, task, and pipeline were under the same project name. i'm seeing what happens if the dataset project name was different ( f"{project_name}_data"
). which project would get deleted... the dataset one or the project of the task that kicked it off?
and the answer is...
the project is preserved, the dataset's project hidden.
so ... empty dataset names due to a small typo in parameter override + the choice for the dataset to have the same project name as the task that created it (...
i think we may have found the frankenbug?
the argument to the dataset name was not being overridden correctly (mistyped), so the default value of an empty string (instead of a placeholder like "CHANGE_ME") in the parent task caused the dataset to basically get created with an empty name, and somehow that hid the whole project, despite hundreds of existing tasks in it.
and no way to un-hide it as far as I can tell?
tasks that create pipelines feels like a hack and i found they dont show up in the UI (have to use the link in the console).
I've found that sometimes i need to right click "Run" a couple of times before the parameters are filled in properly.
Oh neat! I want to take a look at this. Only a few more weeks at the client but it’d be nice to reduce the complexity of the software stack if I can before handoff.
Can you please elaborate on the latter point? My jupyterhub’s fully containerized and allows users to select their own containers (from a list i built) at launch, and launch multiple containers at the same time, not sure I follow how toes are stepped on.
I'm guessing this is done through code-server?
I'm currently rolling a JupyterHub instance (multiuser, with codeserver inside) on the same machine as clearml-server. That’s where tasks are executed etc. so, all browser dev env.
It sounds like there’s an option to basically bypass this latter step and just use clearml’s credentialing to accomplish much the same thing? Am I understanding clearml-session correctly?
if you commit but do not push, the metadata tells clearml that it needs to pull a non-existant commit. any changes you made on top may be saved as a diff, but they'd fail to apply.
for clearml to work on un-pushed commits, it'd have to wait for a push to register a new diff target, which can become a problem (what if you have multiple remotes? which one will it wait for?) so rather, it assumes it can access the most recent commit from your remote repo, and records this as the "base" upon whi...
Oh yes. I see. Yeah, no ML here actually (doing the testing infra of endpoints), but certainly when there is its an issue.
How does clearml session avoid it? I guess only if autoscaling is used (one worker one machine)?
so when the task completed successfully (changed the queue to default and let it finish instead of aborting), the project disappeared.
I ran into something similar during deployment. Hopefully this helps with your debugging: if the agent was launched separately from the rest of the stack, it may not have proper docker-DNS resolution to None . (e.g. if in the same docker-compose, perhaps you didnt add the backend
network field, or if it was launched separately through docker run
without an explicit external network defined)
if the agent's on the same machine, try docker network connect
to add...
dug deeper. if i'm to make a guess.../root/clearml.conf
-> used on startup of agent-services as a template of sorts to create .clearml_agent.<id>.cfg
on demand -> this task-specific file is used to mount to /tmp/clearml_default.conf
in a new container (docker in docker bc of the socket mounted to the agent-services) -> used to execute the task
the project wasn't hidden before. I'm aware of the pipeline tasks being hidden, that makes sense for organization. but the actual project itself as an entirety has a ghost icon.
she created a new project and started working in there, it was visible in the UI... and just now it disappeared again. it's kind of like running the pipeline makes it disappear.
probably, but the syntax would be in that of a git diff, so it’d be a touch clunky if you asked me
Are you trying to avoid local development?
you can put task.execute_remotely() to create it in draft mode. I've taken to configuring defaults to run things very quickly just in case i forget though (e.g. placeholder string for dataset, bail out early if not changed… or just do one epoch on a small subset of samples, etc).
I tried mounting a config file (in the structure of the one on github but with just the relevant s3 section) into the agent-services container at /root/clearml.conf
and after restarting the container, it seems to have had an impact. thank you!
When I inspect the console of the task I'm trying to run, I see there's a call to cp /tmp/clearml.conf ~/default_clearml.conf
in the docker command and that the volume /tmp/clearml.conf
is picked up from the host at some custom-named file ...
I opened github.com/allegroai/clearml/pull/1083 as an attempt to help catch this.
my approach was to spin up an EC2 and run the deployment there from within the EBS volume mount.
I symlinked /opt/clearml
to /mnt/xvda/clearml
to minimize docker-compose changes. been working out fine so far.
with aws-cdk, the deployment steps can be automated (format the volume, clone a repo with the config, etc). I can link you to a resource that may help with that if you're interested.
For reproducibility, it kind of makes sense though. The existence of the file is contingent on the worker cloning the source code. I'm sure things can be done to maintain state differently but I personally adapted to the git-based workflow for managing files pretty quickly.
though yes I will admit I had the same thought first: why must I run it each time?
Beware: squash merges will ruin the ability to reproduce the experiment at that time since the git commit will be lost (presuming th...
Yup if you scroll through the logs in the console, near the top (post config dump), you’ll see a git clone and checkout to the specific hash.
PS You can actually change this parameter in an experiment’s configuration if it is in draft mode.
credentials for the server to do things with s3 will be in /opt/clearml/apiserver.conf.
I think you’d have to run the cleanup service. That’s what seems to be what is controlling deletion based on archived status and some other temporal filters
the clearml github, search for a file named cleanup service dot py (or something to that effect)
I believe pipe.connect_configuration
is what you're looking for?
waiting now to see if they disappear.
any problems you may have spotted with the versions used?
project hasn't disappeared just yet. but it's happened twice now
@<1541954607595393024:profile|BattyCrocodile47> put together None