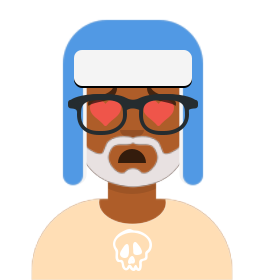
VivaciousPenguin66
17
Questions,
107
Answers
Active since 10 January 2023
Last activity
10 months ago
Reputation
0
Badges 1
93 Γ Eureka!I have just installed the PYPI version of clearml-serving and I get the following error at the command line. clearml-serving --help clearml-serving - CLI for...
3 years ago
///[Please note, all the below was executed on the command line of the compute node, not the server head node]/// I've been following the example on Keras, b...
3 years ago
I am having an issue publishing a completed model training. The model has been deployed on remote compute, using a docker image, and the datasets have been s...
3 years ago
This wasn't a big deal, but I noticed when pushing a dataset to the server, with cloud storage, that the upload information looked a bit bonkers in terms of ...
3 years ago
Question when using remote storage blobs (e.g. Azure). I am using it as a output_url location, and it is storing both datasets, and also experiment artefacts...
3 years ago
I have setup a clearml-server running on a Azure VM instance and have used default parameters when it comes to specifying storage locations for data and arte...
3 years ago
I have got experiments training PyTorch networks on a remote compute run by clearml-agent . I am using the Ignite framework to train image classification net...
3 years ago
Does anyone have an example of how to use the services queue to start a load balancer on Azure? Virtual Machine Scale Sets through the Azure Management Pytho...
3 years ago
I have been successfully deploying and training a PyTorch CNN on a clearml-agent managed compute resource and have been testing some the capabilities, includ...
3 years ago
Hi everyone, Does anyone have any pointers on how to make the ClearML-Server web service secure using SSL by setting up NGINX? I have played around with it a...
3 years ago
I was wondering, if I want to use Task.create() instead of Task.init() to create a new experiment object, I am aware that automatic logging will not be done....
3 years ago
Silly question alert...... Really simple one to start with. If I have the more or less the default settings for a clearml-agent on a compute node, so therefo...
3 years ago
I buried this issue in another thread to do with deployment, but I was wondering if anyone else has had problems using clearml-serving package to serve a PyT...
3 years ago
When I setup my local virtual environment I use a combination of Conda and pip. I use conda as my environment manager, and then use pip for packages that are...
3 years ago
Are there any tips for how to set these boxes in the profile for access to Azure Blob Storage using SAS? I can create a Shared Access Key (SAS) through the A...
3 years ago
I have built a custom docker image and execution script so that I can use Conda as the package manager when installing python packages for job execution. Eve...
3 years ago