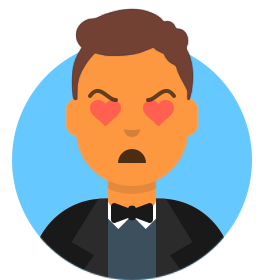
Reputation
Badges 1
606 × Eureka!Very nice!
Maybe for the long-term future you could look into how to make better use of vertical space. Currently, there are 7 (5 in fullscreen mode)= different sections from content to top of the page. Maybe a compact mode would be nice or less space for content headlines.
Now trying changing the default file server.
Is this really working for you guys? I have no clue what's wrong. Seems so unlikely that my code works with artifacts, datasets, but not logging...
AgitatedDove14 Thank you, that explains it.
Sounds good. I think it is obvious that immutability has to be managed by the user then, but this is not different from not using clearml-data, so not a disadvantage in my opinion.
Seems more like a bug or something is not properly configured on my side.
` # Connecting ClearML with the current process,
from here on everything is logged automatically
task = Task.init(project_name="examples", task_name="artifacts example")
task.set_base_docker(
"my_docker",
docker_arguments="--memory=60g --shm-size=60g -e NVIDIA_DRIVER_CAPABILITIES=all",
)
if not running_remotely():
task.execute_remotely("docker", clone=False, exit_process=True)
timer = Timer()
with timer:
# add and upload Numpy Object (stored as .npz file)
task.upload_a...
I think in the paid version there is this configuration vault, so that the user can pass their own credentials securely to the agent.
@<1576381444509405184:profile|ManiacalLizard2> Just so I understand correctly:
You are saying that in your local, user-specific, clearml.conf you set the api.files_server
, but in your remote, clearml-agent, clearml.conf you left it empty?
@<1576381444509405184:profile|ManiacalLizard2> Yes, exactly. I just didn't know how, but now it is all working 🙂
And yes, I have multiple credentials in the clearml.conf of the agents. It's not a good solution, but since I am currently limited to the free version of ClearML, it is the best I could do.
Thanks a lot, now I think I understand.
Debug samples can only be controlled via api.file_server (or programatically)
Could you guide me how to approach this programmatically? Can I implement my own storage adapter for debug samples with ClearML interfaces or am I on my own?
Depends on how you start the task afaik. I think clearml-task uses requirements.txt
by default, but otherwise clearml will parse your files dependencies or if you changed in clearml.conf it will use your conda/pip environment to generate the requirements.
Setting the api.files_server:
s3://myhost:9000/clearml in clearml.conf
works!
@<1576381444509405184:profile|ManiacalLizard2> Thank you, but afaik this only works locally and not if you run your task on a clearml-agent!
I am getting permission errors when I try to use the clearml-agent with docker containers. The .ssh is mounted, but the owner is my local user, so the docker containers root does not seem to have the correct permissions.
Okay, thanks for explaining!
When I add the file the to repo it works fine just like you said.
Yes, I am also talking about agents on different machines. I had two agents on the server machine, which also seem to have been killed. The ones on different machines kept working until 1 or 2 minutes after the clearml-server restarted.
Can you explain what you meant by entropy point file? In a new git repository my code works fine.
Thank you. I am still having the issue. I verified that output_uri
of Task.init works and also clearml-data
with MinIO storage works, but the logger still throws errors
These are the errors I get if I use file_servers without a bucket ( s3://my_minio_instance:9000 )
2022-11-16 17:13:28,852 - clearml.storage - ERROR - Failed creating storage object
Reason: Missing key and secret for S3 storage access (
) 2022-11-16 17:13:28,853 - clearml.metrics - WARNING - Failed uploading to
('NoneType' object has no attribute 'upload_from_stream') 2022-11-16 17:13:28,854 - clearml.storage - ERROR - Failed creating storage object
` Reason: Missing key...
There is no way to create an artifact/model/dataset without a task, right? Just always inherit from the parent task. And if cloned change the user to the user who did the clone.
(just for my own interest: how much does the enterprise version divert from the open source version? It it just extended or are there core changes to the enterprise version)
It is weird though. The task is submitted by the original user and then run on the agent. The task however is still registered by the original user, since it is created by the original user.
Makes more sense to just inherit the user from the task than from the agent?
Okay, this seems to work fine.