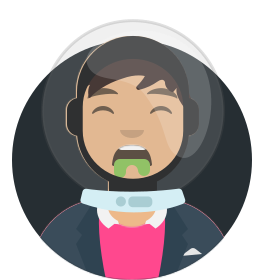
Reputation
Badges 1
92 × Eureka!no. I set apo.file_server to the None in Both the remote agent clearml.conf and my local clearml.conf
In which case, both case where the code is ran from local or remote, will store metrics to cloud storage
@<1523701087100473344:profile|SuccessfulKoala55> I can confirm that v1.8.1rc2 fixed the issue in our case. I manage to reproduce it:
- Do a local commit without pushing
- Create task and queue it
- The queue task failed as expected as the commit is only local
- Push your local commit
- Requeue the task
- Expecting that the task succeeed as the commit is avail: but it fails as the vcs seems to be in weird state from previous failure
- Now with v1.8.1rc2 the issue is solved
right, in which case you want to dynamically change with your code, not with the config file. This is where the Logger.set_default_output_upload come in
If you care about the local destination then you may want to use this None
We use task.export_task()
and a hacked version to get console log:
def save_console_log(task: clearml.Task, fs, remote_path, number_of_reports=10000):
from clearml.backend_api.services import events
from clearml.backend_api import Session
# Stollen from Task.get_reported_console_output()
if Session.check_min_api_version('2.9'):
request = events.GetTaskLogRequest(
task=task.id,
order='asc',
navigate_earlier=True,
...
Are you talking about this: None
It seems to not doing anything aboout the database data ...
1.12.2 because some bug that make fastai lag 2x
1.8.1rc2 because it fix an annoying git clone bug
some clearml cache folder
oh, looks like I need to empty the Installed Package before enqueue the cloned task
what is the difference between vscode via clearml-session and vscode via remote ssh extension ?
For #2: it's a pull rather than a push system: you need to have a script that do pulling at regular interval and need to keep track what new and what not?
@<1523701868901961728:profile|ReassuredTiger98> I found that you an set the file_server
in your local clearml.conf
to your own cloud storage. In our case, we use something like this in our clearml.conf:
api {
file_server: "azure://<account>..../container"
}
All non artifact model are then store in our azure storage. In our self-hosted clearml setup, we don't even have a file server running alltogether
You are using CLEARML_AGENT_SKIP_PYTHON_ENV_INSTALL the wrong way
Artifact can be anything, that you can use clearml SDK to upload to storage. Which storage is used is defined by your clearml.conf (with its credentials) ClearML web and api server do not store those files
Model is a special artifact: None
Example you have the lineage feature where if you train model B using model A as starting point (aka pre-trained) , and model C from model B, ... The lineage will track modelC was built on...
you should be able to use as many agent as you want.
On the same or different queue
Found a trick to have empty Installed package:clearml.Task.force_requirements_env_freeze(force=True,requirements_file="/dev/null")
Not sure if this is the right way or not ...
you may want to share your config (with credential redacted) and the full docker compose start up log ?
We need to focus first on Why is it taking minutes to reach Using env.
In our case, we have a container that have all packages installed straight in the system, no venv in the container. Thus we don't use CLEARML_AGENT_SKIP_PIP_VENV_INSTALL
But then when a task is pulled, I can see all the steps like git clone, a bunch of Requirement already satisfied
.... There may be some odd package that need to be installed because one of our DS is experimenting ... But all that we can see what is...
with
df = pd.DataFrame({'num_legs': [2, 4, 8, 0],
'num_wings': [2, 0, 0, 0],
'num_specimen_seen': [10, 2, 1, 8]},
index=['falcon', 'dog', 'spider', 'fish'])
import clearml
task = clearml.Task.current_task()
task.get_logger().report_table(title='table example', series='pandas DataFrame', iteration=0, table_plot=df)
# logger.report_table(title='table example',series='pandas DataFrame',iteration=0,tabl...
I don't have it so I don't know how things are setup and how to pass on credentials in this case
About the caching: how does it work ? ClearML maintain it own cache and monitor if any of you code changes? Even code that get change inside an import ?
ok, so if git commit or uncommit changes differ from previous run, then the cache is "invalidated" and the step will be run again ?
in my case, I set eveything inside the container, including the agent and not using docker mode altogether.
When my container start, it start the agent inside it in "normal" mode
are you using the agent docker mode ?
you should be able to test your credential first using something like rclone or azure-cli